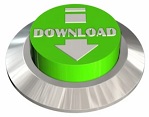
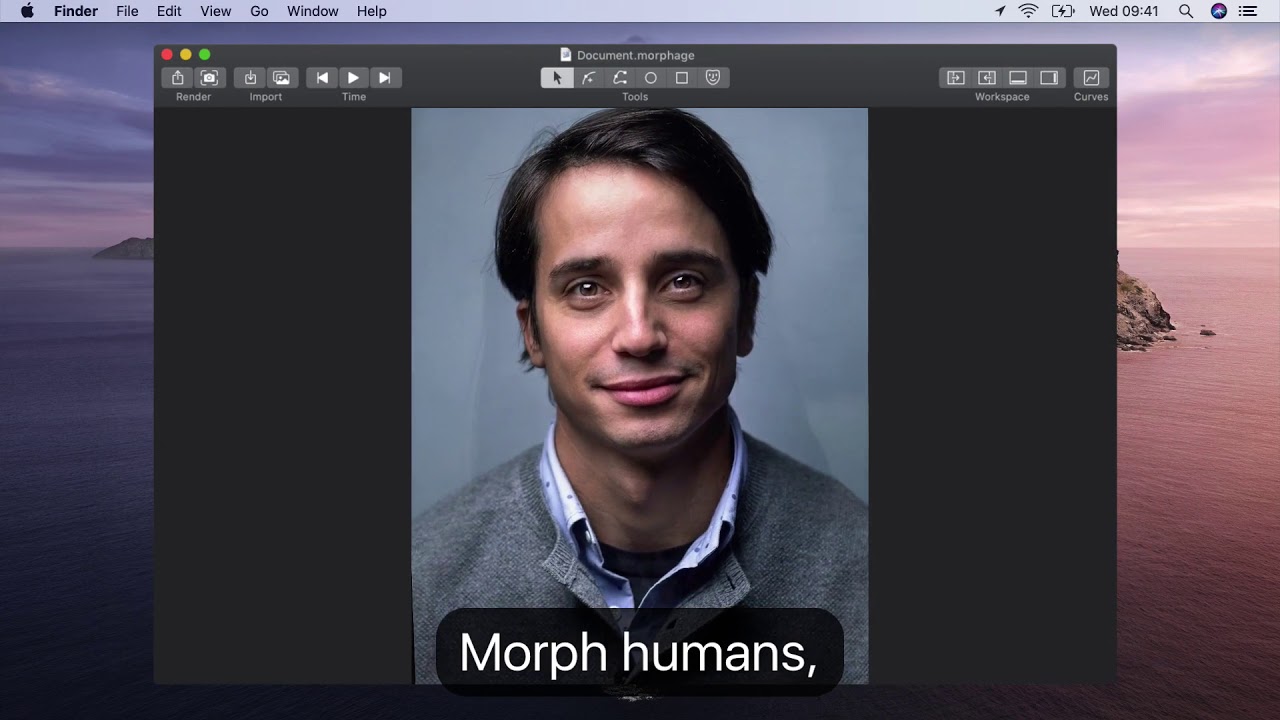
FaceAging.py is a class that builds and initializes the model, and implements training and testing related stuff.From top to bottom, the output faces are in the age ascending order. The first row shows the input faces of different ages, and the other rows show the improvement of the output faces at every other epoch. To achieve better results, it is necessary to train on a large and diverse dataset. Therefore, the saved files are named test_as_male.png and test_as_female.png, respectively. Specifically, the testing faces will be processed twice, being considered as male and female, respectively. Trainingĭone! Results are saved as save/test/test_as_xxx.png Please save and unzip to the folder data. It is better to use aligned and cropped faces. In this demo, we use the UTKFace dataset. You may use any dataset with labels of age and gender. UTKFace (Access from the Github link or the Wiki link).The old version is backed up to the folder old_version.
Face morph age progression sur windows 7 code#
The code is updated to run with Tensorflow 1.7.0, and an initial model is provided to better initialize the network.Please note that you will get errors if running with TensorFlow r1.0 because the definition of input arguments of some functions have changed, e.g., tf.concat and tf.nn.sigmoid_cross_entropy_with_logits.
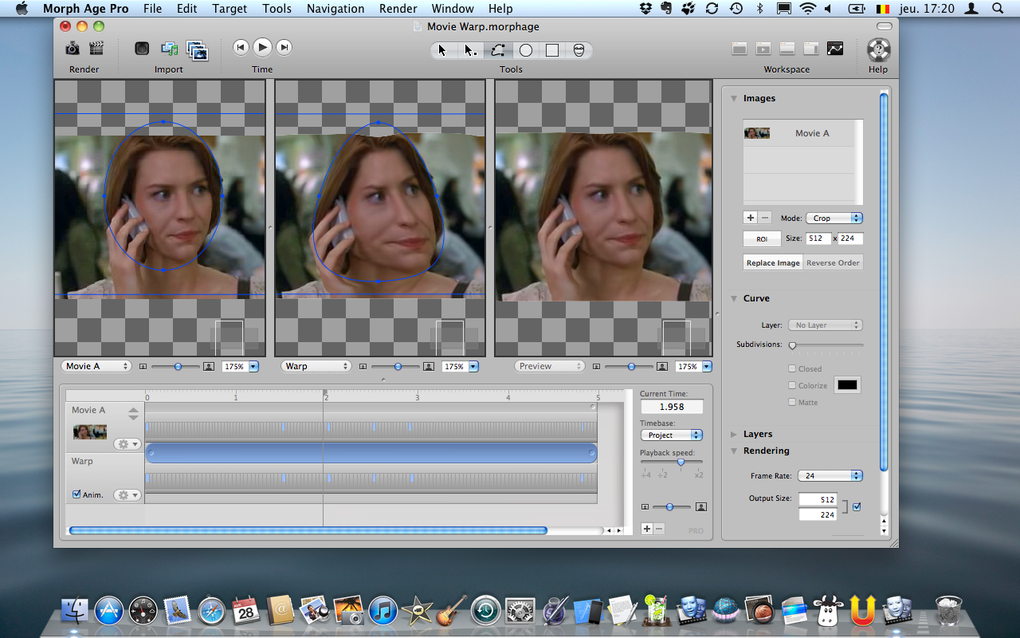
Thanks to the Pytorch implementation by Mattan Serry, Hila Balahsan, and Dor Alt. TensorFlow implementation of the algorithm in the paper Age Progression/Regression by Conditional Adversarial Autoencoder. Age Progression/Regression by Conditional Adversarial Autoencoder (CAAE) | Face-Aging-CAAE Face-Aging-CAAE Age Progression/Regression by Conditional Adversarial Autoencoder View on GitHub Age Progression/Regression by Conditional Adversarial Autoencoder (CAAE)
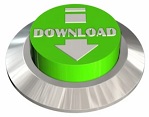